Graph Bayesian Optimization
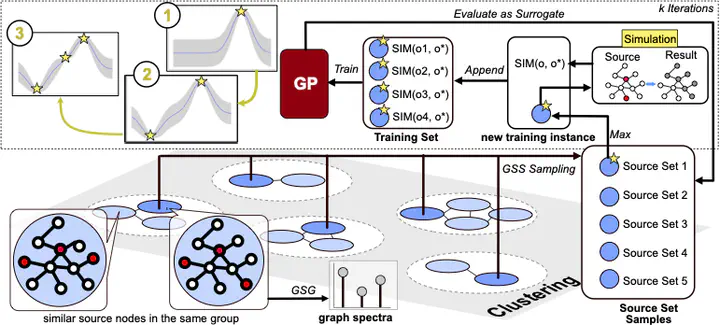
This research aims to provide a coherent framework for generalizing GNNs by bridging the divide between seemingly unrelated works in the spatial and spectral domains, as well as by linking methods within each domain. The study will build a unified theoretical framework that encompasses diverse GNNs. Our research is novel in that it connects disparate GNN models, allowing for direct rethinking and comparison of all GNN models.